Gradient forests: calculating importance gradients on physical predictors
In ecological analyses of species and community distributions there is interest in the nature of their responses to environmental gradients and in identifying the most important environmental variables, which may be used for predicting patterns of biodiversity. Methods such as random forests already exist to assess predictor importance for individual species and to indicate where along gradients abundance changes. However, there is a need to extend these methods to whole assemblages, to establish where along the range of these gradients the important compositional changes occur, and to identify any important thresholds or change points. We develop such a method, called “gradient forest,” which is an extension of the random forest approach. By synthesizing the cross-validated R2 and accuracy importance measures from univariate random forest analyses across multiple species, sampling devices, and surveys, gradient forest obtains a monotonic function of each predictor that represents the compositional turnover along the gradient of the predictor. When applied to a synthetic data set, the method correctly identified the important predictors and delineated where the compositional change points occurred along these gradients. Application of gradient forest to a real data set from part of the Great Barrier Reef identified mud fraction of the sediment as the most important predictor, with highest compositional turnover occurring at mud fraction values around 25%, and provided similar information for other predictors. Such refined information allows for more accurate capturing of biodiversity patterns for the purposes of bioregionalization, delineation of protected areas, or designing of biodiversity surveys.
CITE THIS COLLECTION
SHARE
Usage metrics
Read the peer-reviewed publication
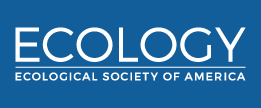
AUTHORS (3)
- NENick EllisSSStephen J. SmithCRC. Roland Pitcher